Aufbau von RAG mit Milvus und Crawl4AI
Crawl4AI liefert blitzschnelles, KI-fähiges Webcrawling für LLMs. Open-Source und für RAG optimiert, vereinfacht es das Scraping mit fortschrittlicher Extraktion und Echtzeitleistung.
In diesem Tutorial zeigen wir Ihnen, wie Sie eine Retrieval-Augmented Generation (RAG)-Pipeline mit Milvus und Crawl4AI erstellen. Die Pipeline integriert Crawl4AI für das Crawling von Webdaten, Milvus für die Vektorspeicherung und OpenAI für die Generierung aufschlussreicher, kontextbezogener Antworten.
Vorbereitung
Abhängigkeiten und Umgebung
Um zu beginnen, installieren Sie die erforderlichen Abhängigkeiten, indem Sie den folgenden Befehl ausführen:
$ pip install -U crawl4ai pymilvus openai requests tqdm
Wenn Sie Google Colab verwenden, müssen Sie eventuell die Runtime neu starten, um die soeben installierten Abhängigkeiten zu aktivieren (klicken Sie auf das Menü "Runtime" am oberen Bildschirmrand und wählen Sie "Restart session" aus dem Dropdown-Menü).
Um crawl4ai vollständig einzurichten, führen Sie die folgenden Befehle aus:
# Run post-installation setup
$ crawl4ai-setup
# Verify installation
$ crawl4ai-doctor
[36m[INIT].... → Running post-installation setup...[0m
[36m[INIT].... → Installing Playwright browsers...[0m
[32m[COMPLETE] ● Playwright installation completed successfully.[0m
[36m[INIT].... → Starting database initialization...[0m
[32m[COMPLETE] ● Database initialization completed successfully.[0m
[32m[COMPLETE] ● Post-installation setup completed![0m
[0m[36m[INIT].... → Running Crawl4AI health check...[0m
[36m[INIT].... → Crawl4AI 0.4.247[0m
[36m[TEST].... ℹ Testing crawling capabilities...[0m
[36m[EXPORT].. ℹ Exporting PDF and taking screenshot took 0.80s[0m
[32m[FETCH]... ↓ https://crawl4ai.com... | Status: [32mTrue[0m | Time: 4.22s[0m
[36m[SCRAPE].. ◆ Processed https://crawl4ai.com... | Time: 14ms[0m
[32m[COMPLETE] ● https://crawl4ai.com... | Status: [32mTrue[0m | Total: [33m4.23s[0m[0m
[32m[COMPLETE] ● ✅ Crawling test passed![0m
[0m
OpenAI API-Schlüssel einrichten
Wir werden in diesem Beispiel OpenAI als LLM verwenden. Sie sollten den OPENAI_API_KEY als Umgebungsvariable vorbereiten.
import os
os.environ["OPENAI_API_KEY"] = "sk-***********"
Vorbereiten des LLM und des Einbettungsmodells
Wir initialisieren den OpenAI-Client, um das Einbettungsmodell vorzubereiten.
from openai import OpenAI
openai_client = OpenAI()
Definieren Sie eine Funktion zur Erzeugung von Texteinbettungen mit dem OpenAI-Client. Wir verwenden das Modell text-embedding-3-small als Beispiel.
def emb_text(text):
return (
openai_client.embeddings.create(input=text, model="text-embedding-3-small")
.data[0]
.embedding
)
Erzeugen Sie eine Testeinbettung und geben Sie ihre Dimension und die ersten Elemente aus.
test_embedding = emb_text("This is a test")
embedding_dim = len(test_embedding)
print(embedding_dim)
print(test_embedding[:10])
1536
[0.009889289736747742, -0.005578675772994757, 0.00683477520942688, -0.03805781528353691, -0.01824733428657055, -0.04121600463986397, -0.007636285852640867, 0.03225184231996536, 0.018949154764413834, 9.352207416668534e-05]
Crawlen von Daten mit Crawl4AI
from crawl4ai import *
async def crawl():
async with AsyncWebCrawler() as crawler:
result = await crawler.arun(
url="https://lilianweng.github.io/posts/2023-06-23-agent/",
)
return result.markdown
markdown_content = await crawl()
[INIT].... → Crawl4AI 0.4.247
[FETCH]... ↓ https://lilianweng.github.io/posts/2023-06-23-agen... | Status: True | Time: 0.07s
[COMPLETE] ● https://lilianweng.github.io/posts/2023-06-23-agen... | Status: True | Total: 0.08s
Verarbeiten der gecrawlten Inhalte
Um den gecrawlten Inhalt für das Einfügen in Milvus handhabbar zu machen, verwenden wir einfach "# ", um den Inhalt zu trennen, was den Inhalt jedes Hauptteils der gecrawlten Markdown-Datei grob trennen kann.
def split_markdown_content(content):
return [section.strip() for section in content.split("# ") if section.strip()]
# Process the crawled markdown content
sections = split_markdown_content(markdown_content)
# Print the first few sections to understand the structure
for i, section in enumerate(sections[:3]):
print(f"Section {i+1}:")
print(section[:300] + "...")
print("-" * 50)
Section 1:
[Lil'Log](https://lilianweng.github.io/posts/2023-06-23-agent/<https:/lilianweng.github.io/> "Lil'Log \(Alt + H\)")
* |
* [ Posts ](https://lilianweng.github.io/posts/2023-06-23-agent/<https:/lilianweng.github.io/> "Posts")
* [ Archive ](https://lilianweng.github.io/posts/2023-06-23-agent/<h...
--------------------------------------------------
Section 2:
LLM Powered Autonomous Agents
Date: June 23, 2023 | Estimated Reading Time: 31 min | Author: Lilian Weng
Table of Contents
* [Agent System Overview](https://lilianweng.github.io/posts/2023-06-23-agent/<#agent-system-overview>)
* [Component One: Planning](https://lilianweng.github.io/posts/2023...
--------------------------------------------------
Section 3:
Agent System Overview[#](https://lilianweng.github.io/posts/2023-06-23-agent/<#agent-system-overview>)
In a LLM-powered autonomous agent system, LLM functions as the agent’s brain, complemented by several key components:
* **Planning**
* Subgoal and decomposition: The agent breaks down large t...
--------------------------------------------------
Daten in Milvus laden
Erstellen Sie die Sammlung
from pymilvus import MilvusClient
milvus_client = MilvusClient(uri="./milvus_demo.db")
collection_name = "my_rag_collection"
INFO:numexpr.utils:Note: NumExpr detected 10 cores but "NUMEXPR_MAX_THREADS" not set, so enforcing safe limit of 8.
INFO:numexpr.utils:NumExpr defaulting to 8 threads.
Was das Argument von MilvusClient
betrifft:
Das Einstellen von
uri
als lokale Datei, z.B../milvus.db
, ist die bequemste Methode, da sie automatisch Milvus Lite nutzt, um alle Daten in dieser Datei zu speichern.Wenn Sie große Datenmengen haben, können Sie einen leistungsfähigeren Milvus-Server auf Docker oder Kubernetes einrichten. Bei dieser Einrichtung verwenden Sie bitte die Server-Uri, z. B.
http://localhost:19530
, alsuri
.Wenn Sie Zilliz Cloud, den vollständig verwalteten Cloud-Service für Milvus, verwenden möchten, passen Sie
uri
undtoken
an, die dem öffentlichen Endpunkt und dem Api-Schlüssel in Zilliz Cloud entsprechen.
Prüfen Sie, ob die Sammlung bereits existiert und löschen Sie sie, wenn dies der Fall ist.
if milvus_client.has_collection(collection_name):
milvus_client.drop_collection(collection_name)
Erstellen Sie eine neue Sammlung mit den angegebenen Parametern.
Wenn wir keine Feldinformationen angeben, erstellt Milvus automatisch ein Standardfeld id
für den Primärschlüssel und ein Feld vector
zum Speichern der Vektordaten. Ein reserviertes JSON-Feld wird verwendet, um nicht schema-definierte Felder und ihre Werte zu speichern.
milvus_client.create_collection(
collection_name=collection_name,
dimension=embedding_dim,
metric_type="IP", # Inner product distance
consistency_level="Strong", # Strong consistency level
)
Daten einfügen
from tqdm import tqdm
data = []
for i, section in enumerate(tqdm(sections, desc="Processing sections")):
embedding = emb_text(section)
data.append({"id": i, "vector": embedding, "text": section})
# Insert data into Milvus
milvus_client.insert(collection_name=collection_name, data=data)
Processing sections: 0%| | 0/18 [00:00<?, ?it/s]INFO:httpx:HTTP Request: POST https://api.openai.com/v1/embeddings "HTTP/1.1 200 OK"
Processing sections: 6%|▌ | 1/18 [00:00<00:12, 1.37it/s]INFO:httpx:HTTP Request: POST https://api.openai.com/v1/embeddings "HTTP/1.1 200 OK"
Processing sections: 11%|█ | 2/18 [00:01<00:11, 1.39it/s]INFO:httpx:HTTP Request: POST https://api.openai.com/v1/embeddings "HTTP/1.1 200 OK"
Processing sections: 17%|█▋ | 3/18 [00:02<00:10, 1.40it/s]INFO:httpx:HTTP Request: POST https://api.openai.com/v1/embeddings "HTTP/1.1 200 OK"
Processing sections: 22%|██▏ | 4/18 [00:02<00:07, 1.85it/s]INFO:httpx:HTTP Request: POST https://api.openai.com/v1/embeddings "HTTP/1.1 200 OK"
Processing sections: 28%|██▊ | 5/18 [00:02<00:06, 2.06it/s]INFO:httpx:HTTP Request: POST https://api.openai.com/v1/embeddings "HTTP/1.1 200 OK"
Processing sections: 33%|███▎ | 6/18 [00:03<00:06, 1.94it/s]INFO:httpx:HTTP Request: POST https://api.openai.com/v1/embeddings "HTTP/1.1 200 OK"
Processing sections: 39%|███▉ | 7/18 [00:03<00:05, 2.14it/s]INFO:httpx:HTTP Request: POST https://api.openai.com/v1/embeddings "HTTP/1.1 200 OK"
Processing sections: 44%|████▍ | 8/18 [00:04<00:04, 2.29it/s]INFO:httpx:HTTP Request: POST https://api.openai.com/v1/embeddings "HTTP/1.1 200 OK"
Processing sections: 50%|█████ | 9/18 [00:04<00:04, 2.20it/s]INFO:httpx:HTTP Request: POST https://api.openai.com/v1/embeddings "HTTP/1.1 200 OK"
Processing sections: 56%|█████▌ | 10/18 [00:05<00:03, 2.09it/s]INFO:httpx:HTTP Request: POST https://api.openai.com/v1/embeddings "HTTP/1.1 200 OK"
Processing sections: 61%|██████ | 11/18 [00:06<00:04, 1.68it/s]INFO:httpx:HTTP Request: POST https://api.openai.com/v1/embeddings "HTTP/1.1 200 OK"
Processing sections: 67%|██████▋ | 12/18 [00:06<00:04, 1.48it/s]INFO:httpx:HTTP Request: POST https://api.openai.com/v1/embeddings "HTTP/1.1 200 OK"
Processing sections: 72%|███████▏ | 13/18 [00:07<00:02, 1.75it/s]INFO:httpx:HTTP Request: POST https://api.openai.com/v1/embeddings "HTTP/1.1 200 OK"
Processing sections: 78%|███████▊ | 14/18 [00:07<00:01, 2.02it/s]INFO:httpx:HTTP Request: POST https://api.openai.com/v1/embeddings "HTTP/1.1 200 OK"
Processing sections: 83%|████████▎ | 15/18 [00:07<00:01, 2.12it/s]INFO:httpx:HTTP Request: POST https://api.openai.com/v1/embeddings "HTTP/1.1 200 OK"
Processing sections: 89%|████████▉ | 16/18 [00:08<00:01, 1.61it/s]INFO:httpx:HTTP Request: POST https://api.openai.com/v1/embeddings "HTTP/1.1 200 OK"
Processing sections: 94%|█████████▍| 17/18 [00:09<00:00, 1.92it/s]INFO:httpx:HTTP Request: POST https://api.openai.com/v1/embeddings "HTTP/1.1 200 OK"
Processing sections: 100%|██████████| 18/18 [00:09<00:00, 1.83it/s]
{'insert_count': 18, 'ids': [0, 1, 2, 3, 4, 5, 6, 7, 8, 9, 10, 11, 12, 13, 14, 15, 16, 17], 'cost': 0}
RAG erstellen
Abrufen von Daten für eine Abfrage
Geben wir eine Abfragefrage zu der gerade gecrawlten Website an.
question = "What are the main components of autonomous agents?"
Suchen Sie nach der Frage in der Sammlung und rufen Sie die semantischen Top-3-Treffer ab.
search_res = milvus_client.search(
collection_name=collection_name,
data=[emb_text(question)],
limit=3,
search_params={"metric_type": "IP", "params": {}},
output_fields=["text"],
)
INFO:httpx:HTTP Request: POST https://api.openai.com/v1/embeddings "HTTP/1.1 200 OK"
Werfen wir einen Blick auf die Suchergebnisse der Abfrage
import json
retrieved_lines_with_distances = [
(res["entity"]["text"], res["distance"]) for res in search_res[0]
]
print(json.dumps(retrieved_lines_with_distances, indent=4))
[
[
"Agent System Overview[#](https://lilianweng.github.io/posts/2023-06-23-agent/<#agent-system-overview>)\nIn a LLM-powered autonomous agent system, LLM functions as the agent\u2019s brain, complemented by several key components:\n * **Planning**\n * Subgoal and decomposition: The agent breaks down large tasks into smaller, manageable subgoals, enabling efficient handling of complex tasks.\n * Reflection and refinement: The agent can do self-criticism and self-reflection over past actions, learn from mistakes and refine them for future steps, thereby improving the quality of final results.\n * **Memory**\n * Short-term memory: I would consider all the in-context learning (See [Prompt Engineering](https://lilianweng.github.io/posts/2023-06-23-agent/<https:/lilianweng.github.io/posts/2023-03-15-prompt-engineering/>)) as utilizing short-term memory of the model to learn.\n * Long-term memory: This provides the agent with the capability to retain and recall (infinite) information over extended periods, often by leveraging an external vector store and fast retrieval.\n * **Tool use**\n * The agent learns to call external APIs for extra information that is missing from the model weights (often hard to change after pre-training), including current information, code execution capability, access to proprietary information sources and more.\n\n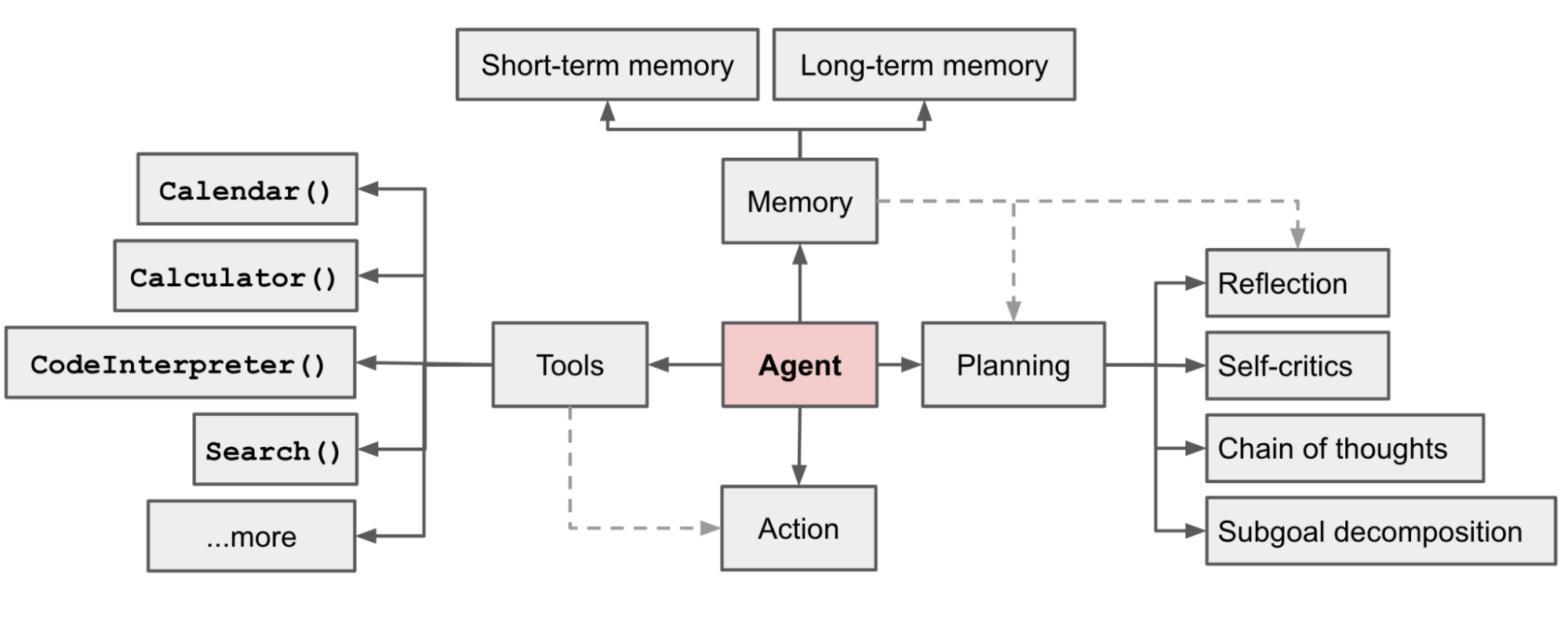 Fig. 1. Overview of a LLM-powered autonomous agent system.",
0.6433743238449097
],
[
"LLM Powered Autonomous Agents \nDate: June 23, 2023 | Estimated Reading Time: 31 min | Author: Lilian Weng \nTable of Contents\n * [Agent System Overview](https://lilianweng.github.io/posts/2023-06-23-agent/<#agent-system-overview>)\n * [Component One: Planning](https://lilianweng.github.io/posts/2023-06-23-agent/<#component-one-planning>)\n * [Task Decomposition](https://lilianweng.github.io/posts/2023-06-23-agent/<#task-decomposition>)\n * [Self-Reflection](https://lilianweng.github.io/posts/2023-06-23-agent/<#self-reflection>)\n * [Component Two: Memory](https://lilianweng.github.io/posts/2023-06-23-agent/<#component-two-memory>)\n * [Types of Memory](https://lilianweng.github.io/posts/2023-06-23-agent/<#types-of-memory>)\n * [Maximum Inner Product Search (MIPS)](https://lilianweng.github.io/posts/2023-06-23-agent/<#maximum-inner-product-search-mips>)\n * [Component Three: Tool Use](https://lilianweng.github.io/posts/2023-06-23-agent/<#component-three-tool-use>)\n * [Case Studies](https://lilianweng.github.io/posts/2023-06-23-agent/<#case-studies>)\n * [Scientific Discovery Agent](https://lilianweng.github.io/posts/2023-06-23-agent/<#scientific-discovery-agent>)\n * [Generative Agents Simulation](https://lilianweng.github.io/posts/2023-06-23-agent/<#generative-agents-simulation>)\n * [Proof-of-Concept Examples](https://lilianweng.github.io/posts/2023-06-23-agent/<#proof-of-concept-examples>)\n * [Challenges](https://lilianweng.github.io/posts/2023-06-23-agent/<#challenges>)\n * [Citation](https://lilianweng.github.io/posts/2023-06-23-agent/<#citation>)\n * [References](https://lilianweng.github.io/posts/2023-06-23-agent/<#references>)\n\n\nBuilding agents with LLM (large language model) as its core controller is a cool concept. Several proof-of-concepts demos, such as [AutoGPT](https://lilianweng.github.io/posts/2023-06-23-agent/<https:/github.com/Significant-Gravitas/Auto-GPT>), [GPT-Engineer](https://lilianweng.github.io/posts/2023-06-23-agent/<https:/github.com/AntonOsika/gpt-engineer>) and [BabyAGI](https://lilianweng.github.io/posts/2023-06-23-agent/<https:/github.com/yoheinakajima/babyagi>), serve as inspiring examples. The potentiality of LLM extends beyond generating well-written copies, stories, essays and programs; it can be framed as a powerful general problem solver.",
0.5462194085121155
],
[
"Component One: Planning[#](https://lilianweng.github.io/posts/2023-06-23-agent/<#component-one-planning>)\nA complicated task usually involves many steps. An agent needs to know what they are and plan ahead.\n#",
0.5223420858383179
]
]
LLM verwenden, um eine RAG-Antwort zu erhalten
Konvertieren Sie die abgerufenen Dokumente in ein String-Format.
context = "\n".join(
[line_with_distance[0] for line_with_distance in retrieved_lines_with_distances]
)
Definieren Sie System- und Benutzer-Prompts für das Lanage Model. Diese Eingabeaufforderung wird mit den abgerufenen Dokumenten von Milvus zusammengestellt.
SYSTEM_PROMPT = """
Human: You are an AI assistant. You are able to find answers to the questions from the contextual passage snippets provided.
"""
USER_PROMPT = f"""
Use the following pieces of information enclosed in <context> tags to provide an answer to the question enclosed in <question> tags.
<context>
{context}
</context>
<question>
{question}
</question>
"""
Verwenden Sie OpenAI ChatGPT, um eine Antwort basierend auf den Prompts zu generieren.
response = openai_client.chat.completions.create(
model="gpt-4o",
messages=[
{"role": "system", "content": SYSTEM_PROMPT},
{"role": "user", "content": USER_PROMPT},
],
)
print(response.choices[0].message.content)
INFO:httpx:HTTP Request: POST https://api.openai.com/v1/chat/completions "HTTP/1.1 200 OK"
The main components of autonomous agents are:
1. **Planning**:
- Subgoal and decomposition: Breaking down large tasks into smaller, manageable subgoals.
- Reflection and refinement: Self-criticism and reflection to learn from past actions and improve future steps.
2. **Memory**:
- Short-term memory: In-context learning using prompt engineering.
- Long-term memory: Retaining and recalling information over extended periods using an external vector store and fast retrieval.
3. **Tool use**:
- Calling external APIs for information not contained in the model weights, accessing current information, code execution capabilities, and proprietary information sources.